Bites of Life: Sensational Science and Publication Bias
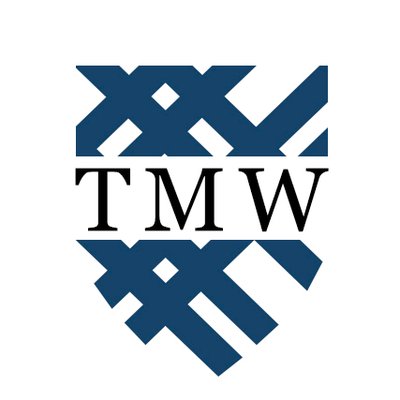
April 7, 2022
Scrolling through nutrition articles, you may stumble across conflicting conclusions. According to studies, coffee both prevents cancer and causes it. Dark chocolate is full of fats and sugar but induces weight loss. Red wine may improve your heart health, while alcohol consumption may increase the risk of high blood pressure, heart failure and stroke. How could scientific studies produce so many contradicting results?
Publication bias is the disproportionate publication of research that discovers new, exciting associations between two variables. This creates incentives to always look for certain results, which may lead to data manipulation or scientific malpractice. Publication bias also distorts the information that the public receives, so that only the most novel or interesting work reaches the press, while more important (or more accurate) work may not make it into journals. This phenomenon heavily contributes to the current reproducibility crisis in science, where poorly conducted scientific studies may give inaccurate results that don’t reflect other scientists’ findings.
According to a Nature survey of 1,576 scientists, over 70% have tried and failed to replicate their own and others’ work. This may be a signal that academia as an institution may need to adjust its structure to better encourage accurate, reproducible work.
In statistics, correlation between two variables is usually measured using a metric called a p-value, which describes the probability of finding a certain result if there is actually no real relationship between the variables of interest. If the p-value of a result is very small, then this could be evidence that two variables are related.
To gather more information, The Mac Weekly spoke to Macalester’s statistics professors and Richard Horton, Editor in Chief of The Lancet, an international medical journal based in the UK.
According to professor Kelsey Grinde, researchers and journals are always chasing positive results, or some sort of statistically significant relationship that has a small p-value.
“A lot of [publication bias] has to do with the way academia and science is structured and the way professors and scientists are evaluated,” Grinde said. “There’s a lot of pressure to publish.The journals get to decide whether a paper is good enough to be in a journal or not. If those people are focused on small p-values, or how novel or interesting a result is, then people are still going to feel that pressure until we change the way we are evaluated.”
This may create incentives for researchers to look for relationships that don’t exist, or to over exaggerate findings.
“Data are not objective and math is not objective, Grinde said. “There are a lot of decisions along the way.”
There are quite a few ways of tinkering with data to find interesting results. Because of the way that p-values are calculated, increasing the sample size could change whether or not a result is considered statistically significant. This is especially true in the age of technology, where collecting and storing data is easier and cheaper.
“We talk a lot about ‘big data,’” Grinde said. “Everyone is collecting data about everybody. The more observations you have, the easier it is to get a small p-value.”
With access to so much data, statisticians can compare lots of variables at once to see if there are any correlations. Once they find one, bingo! They can publish this as a positive result. These results are not necessarily a confirmation that one factor causes another, or even that a real correlation exists.
“If you look at enough things, you can eventually find a small p-value somewhere, by chance.” Grinde said.
Postdoctoral Fellow in statistics Bryan Martin listed other ways to tease out a desired result: including or excluding outliers, weighting variables and including or excluding certain variables can all impact findings.
“You can do anything with enough data,” Martin said. “By obscuring the decisions you make, you can hide a lot of dubious work. If looking at the results influences how you mess with outliers or decide what variables to include, then the results are no longer valid. The p-value no longer represents what the p-value is a measure of. That’s a concept that’s lost on a lot of scientists.”
There are two layers of motivation behind publication bias. From one side, authors and researchers want to discover new medicines, causes of certain reactions and exciting correlations. From the other, journals want to be on the cutting edge of science. For these reasons, both researchers and journals seek positive, statistically significant findings.
According to Martin, even well conducted studies must pass through journal referees and editors to be published, whereupon only positive fundings make it to print.
“If you do an analysis and you find that two things are not correlated, that’s not going to get published because no one cares,” Martin said. “There’s an incentive to always try and produce results, whether they are actually there or not.”
Richard Horton, Editor-in-Chief of The Lancet, said that there are unique pressures in selecting articles for publication.
“Just as the New York Times has to decide what to put on the front page, so do we,” Horton said. “We have to think about what issues are topical, original and will be of interest to our readership. We make deliberate choices about what we publish. If a finding has been published elsewhere, then we probably don’t want to be the second or third journal to publish it. We would like to be the first.”
Apart from the push for novelty, there can also be a disconnect between study results and subsequent press communication of the findings.
“In popular media … There can be a lot of sensationalizing, whether intentionally or unintentionally,” Grinde said. “It’s very easy to put spins on things that will grab attention but maybe aren’t what the study was actually saying. There’s a lot of nuances to what gets published and what gets reported on.”
Horton encouraged scientists to publish null results.
“If you have a null result and you decide not to publish it, then obviously all the journals will be filled with positive results so you do get the bias” Horton said. “Everything we can do to encourage scientists to publish null results is important. This is the best way to tackle publication bias.”
“Even if a study’s p-value isn’t big, we still learn something,” Grinde agreed.
While Grinde and Martin fear that bias may be contributing to greater public distrust in science, Horton claims that publication bias is inevitable, and not necessarily undesirable.
“I’m all in favor of [publication bias] … A journal is not just a neutral repository for scientific papers,” Horton said. “It is a very engaged voice in debate and discussion about whatever field it’s working in. That means that inevitably, journals have opinions. For us, we’re a medical journal so we try to be an active participant in debates about health worldwide, including the political dimensions of health. These opinions are expressed and published every week in editorials, in the news pieces we commission, and in the papers we choose to publish.”
Before publishing, The Lancet critically appraises research with medically qualified editors, and passes articles by field experts and medical statisticians. Despite this, irreproducible results sometimes make it to print.
“I think journals do their best to sanitize the scientific record, but inevitably, unfortunately, bad papers do get through. When a bad paper does get through, it’s important that journals correct the record as quickly as possible,” Horton said.
In order to avoid unreliable science, Kelsey Grinde suggests checking the study for conflicts of interest, and keeping an eye out for sensational or causal language.
“Follow the money,” Grinde said. “And correlation is not causation; if they’re using causal language, be wary of the conclusions they draw.”
Martin suggested checking how many hypotheses the researchers tested and regressions they ran, and ensuring that there are explanations for their methods.
“The good thing about science is that it’s a pretty effective self correcting mechanism, but the self correction only works if we’re willing to admit our own mistakes,” Horton said.